For the last few years I’ve given a talk to incoming Ph.D. students in Molecular Biology on why they should consider doing Computational Biology research. I’m fairly passionate about making this pitch, since I strongly believe all 21st century Biologists should have a greater (or lesser) degree of computational training, and that the best time to gain that training is during a Ph.D. or a Post-Doc.
I’ve decided to post an expanded version of the reasons I give for why Biology trainees should gain computational skills in hopes of encouraging a wider audience to consider a research path in Computational Biology. For simplicity, I define the field of Computational Biology to include Bioinformatics as well, although there are important distinctions between these two disciplines. Also, I note that this list is geared towards convincing students with a background in Molecular Biology to consider moving into Computational Biology, but core aspects and variants of the arguments here should apply to people with backgrounds in other disciplines (e.g. Ecology, Neuroscience) as well. Here we go…
0. Computing is the key skill set for 21st century biology: As time progresses, Biology is becoming a more quantitative science. Over the last three centuries, biology has transformed from an observational science into an experimental science into a data science. As the low-hanging fruit gets picked, fundamental discoveries are getting harder to make using observation and experiment alone. In the future, new discoveries will require leveraging big datasets and using advanced analytical methods. Big data and complex models require computational skills. Full stop. There is no way to escape this reality.
But if you don’t take my word for it, listen to what Nobel-prize winning pioneer of molecular biology Walter Gilbert, who made this same argument about the future of biology over 20 years ago:
To use this flood of [sequence] knowledge, which will pour across the computer networks of the world, biologists not only must become computer literate, but also change their approach to the problem of understanding life.
Or listen to Nobel-prize winning pioneer of molecular biology Sydney Brenner, who has been banging on about this issue for years:
I spent many hours persuading people that computing was not only going to be the essential tool for biological research but would also provide models for analyzing complexity…The development of sequencing techniques and their widespread application has generated enormous databases of information, and the need for computers is no longer questioned
1. Computational skills are highly transferable: Let’s face it, not everyone doing a Ph.D. or Post-Doc. in Biology is going to go on to a career in academic research. The Washington Post recently reported that “only 14 percent of those with a Ph.D. in biology and the life sciences now land a coveted academic position within five years“. So if there is high probability that your Ph.D. or Post-Doc training will need to be used outside of academic research, why not acquire the most broadly applicable skill set that you can? Experimental skills only transfer to laboratory jobs in the biosciences or medical job market. Computational skills transfer across this sector, plus a much wider market outside of the (bio)science. Increasing your computational chops won’t just give you a better chance at landing a job. It will have added benefits in your own life as well, since you will have a deeper appreciation for how computers work and more mastery of when you interact with computers in your daily life.
2. Computing will help improve your core scientific skills: Biology is inherently a messy subject. While some Biologists are rigorously trained in how to cope with this messiness through good experimental design and statistical analysis (here’s looking at you my Ecologist sisters and brothers), the sad truth is that many (most?) Biologists have bad habits when it comes to data collection and analysis. Computing forces you to confront and tame the very human tendency to do science in ad hoc ways and therefore it naturally develops core scientific skills such as: logically planning experiments, collecting data consistently, developing reproducible methodology, and analysing your data with proper statistical methodology. So even if you can’t be convinced to abandon the bench or field forever, computational training will develop scientific best-practice that crosses-over and enhances your experimental skills set.
3. You should use you Ph.D./Post-Doc to develop new skills: Most Biologists come into their Ph.D. with some experimental training from high school and undergraduate studies. OK, so maybe this training isn’t cutting edge and you haven’t done advanced research to really hone your experimental skills, but nevertheless you do have some amount of training under your belt. In contrast, the vast majority of Biology Ph.D. students have no training in scientific computing skills beyond using Excel or a GUI-based statistics package. So use your Ph.D. or Post-Doc. time to for what it should be — training in something new, not just further developing a skill set that you already have.
My view is that the best time to train in Computational Biology is during a Ph.D., and the last chance to do this is likely to be as a Post-Doc. This is because during your Ph.D. you have time, secure funding and a departmental structure to protect you that you will never have again in your career. Gaining computational skills as a Post-Doc is also a great option, but shorter contracts, greater PI dependency, and higher expectations to publish mean that you typically don’t have as much time to re-train as you would during a Ph.D. Good luck finding the time to re-tool as a PI.
4. You will develop a more unique skill set in Biology: As noted above, the vast majority of Biologists have experimental training, but very few have advanced Computational training. While this is (thankfully!) changing, you will still be at a competitive advantage for at least a decade or more in terms of getting results in post-genomic Biology if you can code. And because you will be able to get results that many others cannot, plus the fact that you will have skills that set you apart from the herd, you will be more competitive on the job market.
5. You will publish more papers: While it may not always feel like it, a Ph.D. or Post-Doc goes by quickly. Therefore, you don’t have a lot of time to waste time with experiments that fail, if you want to stay in the game. Don’t get me wrong, Computational Biology will provide you more than your fair share of failed experiments, but crucially they will fail in hours/days instead of weeks/months, and therefore allow you to move on to something that works more quickly. As a result, you are very likely to publish more papers per unit time in Computational Biology. Whether you believe the old chestnut that experimental papers are somehow “harder” and therefore have more worth (I don’t), it is clear that publication remains the hard currency of science. Moreover, the adage that search committees “know how to count even if they can’t read” is still as true as ever. More seriously, what employers and funding agencies want to see is junior researchers who have good ideas and can take them to completion. Publication is the proof that you can finish projects. Computational Biology will allow you to demonstrate that you are a finisher, and that you have what it takes to succeed in science, a little bit faster than the next person.
6. You will have more flexibility in your research: I would say one of the greatest thing about being a Computational Biologist is that you are not as constrained in your research as you are when you do Experimental Biology. Sure, you can only work on projects that are amenable to computational analysis, but this scope is vast — from Computational Neuroscience to Theoretical Ecology and anything and everything in between. You can also move from flexibly from topic to topic more easily than you can if your skill set is linked to specific experimental techniques. This flexibility in scope allows you to satisfy your intellectual curiosity or chase the latest trend as you wish. Most importantly for trainees, the flexibility (and low-cost, see below) afforded by Computational Biology research allows you to make the case to your PI to develop your own research programme earlier in your career. This is crucial since the more experience you have designing independent projects early in your career, the more likely you will be to succeed if/when you make it to the big time.
7. You will have more flexibility in working practices: ‘Nuff said:
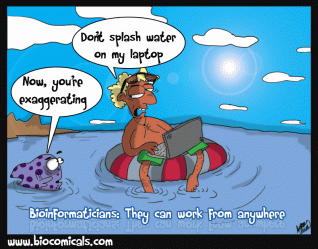
Seriously though, Computational Biology has many pluses when it come to balancing work and life, but still maintaining a high level of productivity. Unlike being chained to the bench, you can do Computational Biology from pretty much anywhere, and telecommuting/working from home are standard practices in Computational Biology. Over the longer term, this flexibility in work practice helps you to accommodate career-breaks, manage the tough times life will throw at you, and make big life decisions like starting a family easier, since you can integrate coding and submitting jobs to the cluster into your life much better than you can integrate racing back to the lab to flip stocks or harvest cells. Let me say it loud and clear right here: if you want to have a career in academic Biological research and also have a family, choosing to do a Ph.D or Post-Doc in Computational Biology will be more likely to get you to this goal than if you are stuck in the lab. This is not just true for women, as I and others can attest to:
8. Computational research is cost-effective: With the wealth of publicly available data now available, Computational Biology research is cheaper than most experimental work that requires a large consumables budget. This is important for a number of reasons. Primarily, work in Computational Biology is less dependent on grant funding, and therefore you don’t have to be a slave to trends or waste inordinate time chasing grant funding — you can actually just get on with the job of doing the science you want to do. This is especially important in tough economic times like the present moment. As mentioned above, the reduced cost of Computational Biology research also allows trainees to design their own research at an earlier career stage, since you will not be as reliant on a PI to authorize expenditure for your project. Cost-efficiency is also very important when you are starting your group and for maintaining continuity of productivity when riding out troughs in funding or group size. Finally, the cost-efficiency of Computational Biology allows researchers in developing scientific economies to be on equal parity with researchers in rich countries. In my opinion, trainees from BRICS nations and other developing economies (sorry to use this somewhat judgemental term) should really consider choosing Computational Biology as a way to get to the top of the class globally without being limited by the need for big budgets.
9. A successful scientist ends up in an office: This is the kicker. If you succeed and get that “coveted” PI position, you will ultimately end up stuck in an office. True, some brave souls still find time to make it into the lab to do experiments, but they are a rare breed. The truth is that the native habitat for an academic researchers is sitting in their office in front of their computer. You can’t do a lick of wet lab or field work from the office, but you can still do Computational Biology research from behind a desk! As noted by Webb Miller, one of the most highly-cited bioinformaticians ever, continuing to do your own research is also one of the best ways to stay motivated about your work over the long haul of a career. Remember that the long-term goal is to be a “Principal Investigator”, not an “In Principle Investigator,” so if you’ve really wanted to do research since you were young, then ask yourself: why train in skills you will never ultimately use for the majority of your career, while somebody else in your lab gets to have fun making all the discoveries?
[10. You will understand why lists should start with the number zero.]
A major reason I have for posting this list is to start more discussion about the benefits of doing research in Computational Biology. I have deliberately made this a top N (not a top 10 list) so that good ideas can be added to the above. I’ll update this post with good suggestions from the comments, and give full credit to the originator.